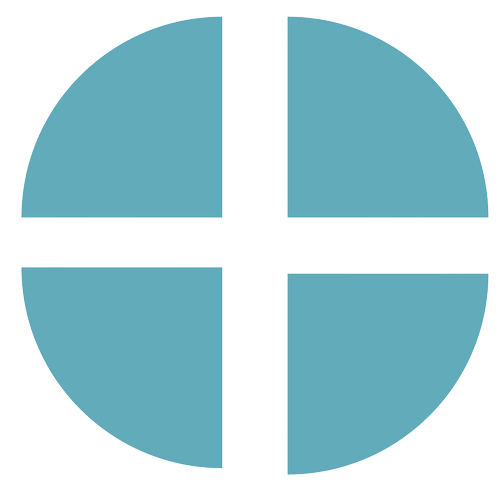
AI in Practice Part 3: Organizational Change
How do you plan for evolving business models, UI/UX, and company culture?
.png)

AI in Practice Series
Part 1: Proprietary Data & Compliance
Part 2: Product Strategy
Part 3: Organizational Change
We are in the implementation phase of AI technology. While hype is fun, we wanted to help executives grapple with the big question: what the hell do you do now?
To figure it out, we put together two round table sessions with 25+ different executives: CEOs, CTOs, CPOs, and AI leads from 20+ companies across a variety of industries, representing a mix of private companies with an aggregate of $30B+ in valuation and $5B+ in funding and several public companies.
We’ve compiled, anonymized, and written down the best insights from those working sessions, and the takeaways are diverse and enlightening. We are entering into a new age of software, and many previous best practices will have to be reconsidered.
In this essay, we will cover evolving business models, UI/UX strategies, and fostering an AI-forward company culture.
Business Model / Pricing
One fundamental decision is which business model to use and how much to charge. The executives we’ve spoken with expect to see business models change as AI becomes introduced into the pricing equation. Per-seat and usage-based pricing will have to evolve as more companies adopt AI and incorporate LLM features.
New Pricing Model: Value-based Pricing
Companies are exploring a new concept of “value-based pricing” for AI products. This idea proposes customers only pay for the value delivered to them.
“Our pricing model is becoming far more value-based. We’ve always been usage-based, but we’re starting to try to use large language models to better measure the value we create for our customers. I think actually, phonetically, that may represent a shift we see in our industry. [...] not only from seat-based pricing to usage-based pricing, but from usage-based pricing to value-based pricing.” - CEO at $1B+ Private Tech Company
But what does that mean and how is it different from existing pricing?
Value-based pricing requires a thorough assessment of your customer’s ROI calculation and how you can best align pricing to the value they get from your product. The per-seat model assumes that each X dollar paid per license generates Y dollars of value per user. That is, if you pay $1K per head for a S&M solution that helps you find leads, the assumption is the value of the leads generated from this solution is greater than the $1K you paid. The consumption model assumes the amount that you “consume” aligns with the value you are getting—you pay $10 per lead generated, assuming the value of each lead will be greater than that $10.
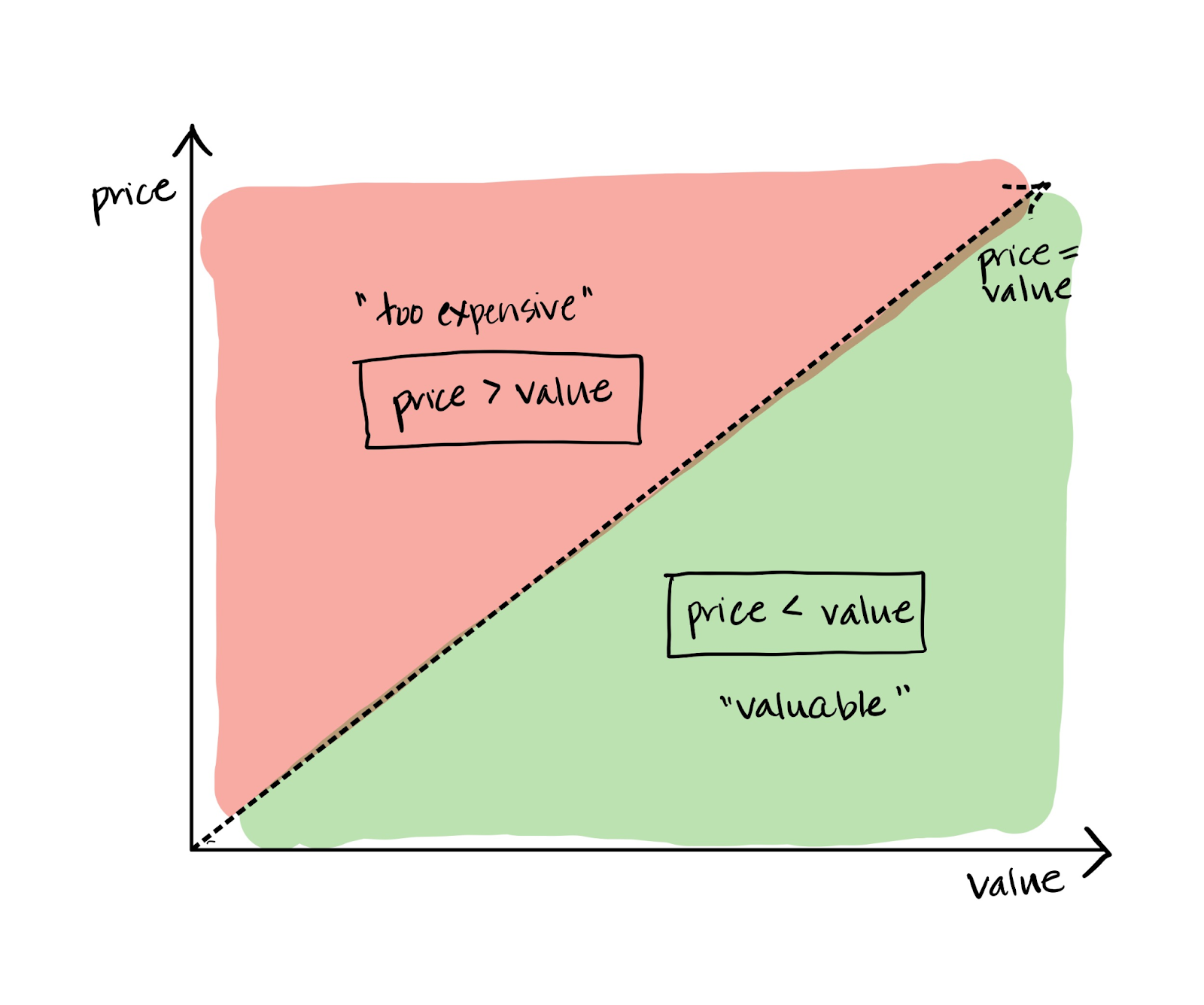
For value-based pricing, you need to first understand where the value is being generated. We often think about technology as making things faster and cheaper, but this might not be what the customer attributes as the “value” they get. It could instead be increasing accuracy, improving customer service, increasing upsell sales, better branding, etc. The better you can align pricing to a customer’s true value, and the better you can make their outcome, the more you can charge.
In our marketing software example, value-based pricing implies that if you receive a lead—the value being whether that lead then becomes a customer or $X of revenue—the vendor then collects Y dollars for generating that revenue.
This may ultimately lead to more enterprise-style pricing with custom terms and value definitions for each customer or customer type. CCC, for example, has leveraged value-based pricing through a charge-per-claim that no longer needs a human placement on their Estimate-STP product.
“Our pricing at the carriers is largely focused on an ROI basis. We look at the value that we drive against our clients and price the products on a return. It could be a 3:1 return, a 5:1 return or a 7:1 return. [...] It's not contingent pricing, but we look at the value that's being driven from the solution and the efficiency it allows, and we set the market price based on that ROI.” - Executive VP, CFO & Chief Administrative Officer at 51st Annual J.P. Morgan Global Tech Conference (May 23, 2023)
EvenUp is another company that has uncovered where their customers find value (accuracy of claim numbers) and then prices off of the subsequent uplift their customers see. They contribute two major pieces of the demand letter: a summary of the personal injury case and a specific dollar amount of compensation that’s getting claimed. Initially, the value was thought to come from writing that letter faster and better than a human can. However, EvenUp found that most of the value comes instead from being more accurate with the dollar estimate.
Applying value-based pricing, EvenUp prices are based on how much uplift they can drive in cases. They combine both the LLMs and a proprietary dataset to drive value that accrues over time. The pricing framework is built on time-saving and better outcomes—the better the outcome EvenUp delivers, the more they can charge.
Defending Upsell
We are also seeing companies use AI features to better defend upgrade seats. Executives are cautiously optimistic about AI’s impact on their premium seat pricing.
“We’re better able to defend some of our upgrade seats through the use of AI. [...] I’m cautiously optimistic that, for some businesses, there may be a way to defend the value of more premium seats through the use of AI.” - CEO at $3B+ Private Tech Company
There is no clear answer to date as to the best strategy. Some companies, like Notion, Grammarly, GitHub, and most notably, Microsoft, have opted to charge a premium for newly released AI features. Others, like Canva, Quizlet, Adobe, and Quora provide certain AI features free of charge to all users, with the rationale that AI enhances their product set and increases engagement. We are starting to see some of these free offerings get tucked into paid tiers or usage volume paywall limits to improve conversion.
Drawing a parallel to the early days of SaaS, we similarly saw a slew of custom pricing and packaging deals. Eventually, we landed on the standard and simple seat-based pricing that exists today (with a recent shift towards usage-based). We are witnessing an analogous evolution in the AI pricing strategy as companies continue to experiment with how to best charge their customers.
Managing Costs
The other piece of the business model to consider is the cost to service and deliver AI features. Building, productionizing, and maintaining these features is no small feat, and the costs can rapidly grow out of control. The advice we’ve heard is to assume a long-term LLM completion cost (what you would expect an LLM-generated response to cost in the future) and manage current AI investments with this long-term cost expectation in mind. This is recommended for two reasons:
- Being too cost-conscious will slow down innovation. For many, the benefits of rapid innovation outweigh the current costs.
- LLM costs are declining and this trend is expected to continue. There will be plenty of future opportunities to optimize costs.
“The strategy that we’ve employed is to pick a long-term LLM completion cost that we think the industry will asymptote to […] and build around that cost. The trade-off I’ve made is that cost consciousness will slow down innovation. If teams anchor on 70% GPT-4 completion, you’re just not going to get the innovation that you want. We also believe that the cost of large language models […] is going to fall quite precipitously. Our strategy has been to focus on innovation and not be too cost-conscious at this time.” - CEO at $1B+ Private Tech Company
AI will change the ways in which you make money off of your customers. Your customers will not only see these changes on their invoices, but they will also see it reflected in the user experiences they’re paying for.
UI/UX Evolution
Building user experiences is a defensive exercise when using LLM-powered features. Mostly, this is about learning how to deal with hallucinations. We’ll discuss a few of the strategies that were shared for managing this risk.
Clear messaging with customers. Companies need to communicate upfront to customers that this is not a perfect product. That way, when the product inevitably generates incorrect outputs, customers will understand this is a known problem associated with the underlying generative AI technology, rather than thinking something has gone wrong with your specific product.
Build defensiveness into the UX. Three things are inevitable. Death, taxes, and users ignoring the instructions. Even when you communicate pitfalls to your users through detailed documentation, users won’t read them, so you’ll need to build guardrails into the UX. What does this mean in the short term? Framing things as suggestions/ideas rather than definitive truths.
The other strategy we’ve seen is using filters (truthfulness, helpfulness, etc.) to manage hallucinations. Pre- and post-generation filters ensure that the generation remains grounded in the knowledge you’ve integrated into the product by refining content to meet predefined criteria. These could be certain guidelines, like avoiding inappropriate or sensitive topics, or maintenance of a particular tone or style. Filters make sure that the generated text is consistent with the desired context and quality.
“In the user experience, you actually need to be defensive. You might say something like, ‘Here’s one random idea. Take it or leave it.’ As opposed to saying, ‘This is the right answer.’” - VP of Product at $100B+ Public Tech Company
Build human touchpoints and feedback loops. Human review and confirmation can be incorporated throughout the AI generation process. This increases the accuracy of the output and reduces the risks associated with generative AI products. More importantly, this creates a vital feedback loop for users to alert you when an answer isn’t right. A combination of these explicit and implicit (data analytics) signals ensures your model and product continue to perform in line with your and your customer’s expectations.
“Beyond the technical strategies for reducing hallucinations to as low a point as possible, it’s really been about building the right human touchpoints into the system [...] Having the human confirm structured data along the way reduces the especially harmful hallucinations, making it into just wrong guesses that the human is happy to discard at the final checkpoint.” - CEO at $3B+ Private Tech Company
Empathetic design. Many of these features are foreign to customers, so the design of your UI/UX should aim to put them at ease. The hype around AI has fed into a sense of insecurity; your product experience should empathize with that feeling and give people a sense of agency. Similar to these executives’ advice on AI culture, which we will discuss shortly, users should view AI as a capable colleague. This framing opens them up to discovering new modes of interaction.
AI automating everything away is a negative experience for many, especially in business processes. For now, users are more comfortable when AI is on the sidelines providing suggestions as a helpful coach that only jumps in when requested. One example we’ve seen of this is introducing AI through a visualization tool. CCC’s Engage customer experience platform shows how AI can support outcomes. The generated damage heatmap gets people’s attention and helps them easily understand where the vehicle damage is. This understanding then leads them to dig deeper into the insights and better embrace AI suggestions, such as labor hour predictions.
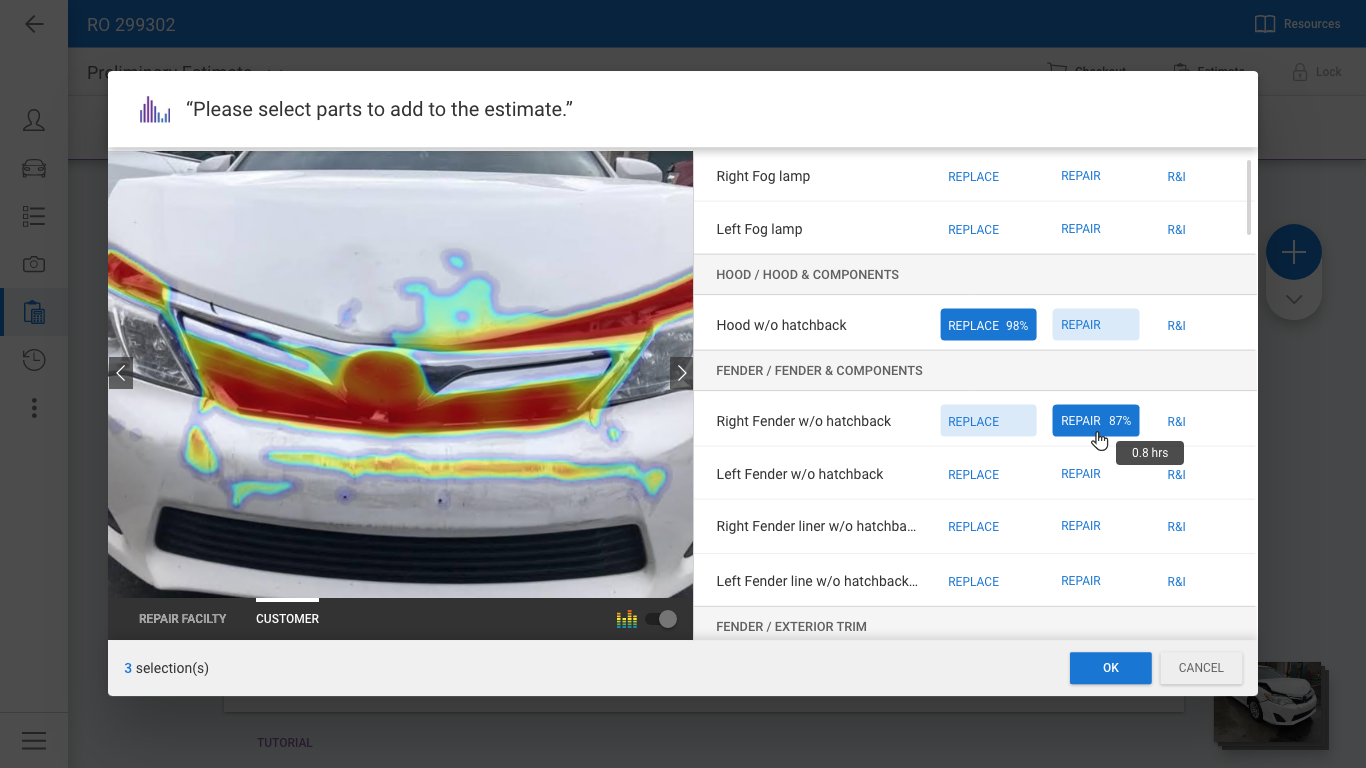
Experiences should not be a significant step change. AI can not instantly render all the workflows your customers have built over the past years obsolete. Your users have developed muscle memory for getting things done on your platform, and the familiarity of routine makes small changes feel uncomfortable and big changes untenable.
In addition, while the unlimited promise of a blank chat box is appealing, it’s not what most people want. While there is value in giving users the ability to ask for what they want in natural language, it can be daunting when you put the onus on them to know what they want and what the software can provide. Flexibility is powerful, but only if your user is prepared. Help your customers figure out what they want while giving them the power to get it. Notion does this well with smart suggestions throughout its product. ChatGPT is a good counterexample—a powerful tool, but one where you have to sift through web articles and Twitter threads to figure out what it can actually do.
Create guardrails through customer approvals. This increases in importance as we move from generative outputs to generative actions (LLMs starting to take actions on your behalf). Consequences related to actions are much greater than text outputs. To mitigate these risks, customers should be involved and give explicit approval at important junction points.
Generative AI has and will change the way we interact with technology. As users’ expectations evolve with increased exposure to new types of UI/UX, the tech companies of today will need to adapt as well.
Culture and Workforce
AI changes rapidly—what is true one day may not be true the next. This is not a new experience for tech companies, for whom there’s always been pressure to stay ahead of the curve. AI multiplies this pressure tenfold. Companies must upgrade their culture to be able to handle the technological whiplash.
The executives we interviewed were deploying the following strategies to stay on top:
- Creative AI experimentation is more important than technical prowess
- Communicate the value of exploring AI collaboration
- Establish guardrails around investments in AI exploration
Creativity over Technical Prowess
Every person, regardless of technical aptitude, has been able to experience the capabilities of generative AI through personal experimentation. The sparks of creativity don’t come exclusively now from a data scientist or ML practitioner, but from individuals across an organization, whether it be a marketer finding a use case for LLMs or engineers jumping headfirst into tinkering with new builds and products.
This creativity is driven by how LLMs have decreased the time to market for products. AI-adjacent projects that would have taken years can now be done in a weekend. API access to models like GPT-4 means that teams no longer need to do the work of training models themselves and can dive right into building applications.
AI ideas won’t only come from the expert with years of research experience or the data scientist with a PhD. They can and should come from your entire workforce experimenting with how ChatGPT or an LLM API could enhance their workflow.
“Technology is [...] ahead of its application right now. The capabilities exceed what we’ve actually discovered at this point. Therefore, there’s a premium not on technical aptitude, but on creative discovery and application of the technology. The biggest returns, I suspect, are not going to come from some data scientist using an LLM working alongside a marketer. It’s going to be from the marketer using the LLM in a creative use case.” - CEO at $1B+ Private Tech Company
Communicate the Value of AI Exploration
Employees need to understand that AI can or will be able to do their job. The new default should be to accept this possibility and find ways to work with it. Once the fear (“It can do everything I can”) and defensiveness (“There is no way it can do everything I can”) fade, the mindset of, “This can replace me,” should be redirected towards, “This is something that works alongside me.”
“You have an AI consultant who is working right next to you. Assume it can do everything you can do, and default to working with it. We’ve started to see, with that sort of mentality, that creativity or experiences accelerate.” - CEO at $1B+ Private Tech Company
Employees need the time and opportunity to figure out how AI will change their jobs. The best way to help them in this discovery process is by celebrating when they do find new ways to work with ChatGPT, and encouraging employees to collaborate with each other. We’ve heard that AI-specific Slack channels and internal hackathons are useful in elevating understanding and increasing creativity in a short period of time.
Looking back, a great example of this phenomenon is what Google did around the Internet paradigm shift. They gave employees 20% of their time just to tinker, even if it didn’t directly relate to their day job. This resulted in some of their most successful products: AdSense and Google News.
Ultimately, AI adoption is not a “yes/no” decision but rather a “when” decision for businesses and employees alike, so fostering this mentality early will pay dividends down the road.
Establish Guardrails
For many companies, the flames of AI enthusiasm don’t need to be stoked but contained. While encouragement of exploration is the north star, the appropriate precautions also need to be put in place.
Early guidelines are crucial to making sure that employees don’t accidentally put data where they shouldn’t (saving you from unsavory headlines). Whether that’s outright banning the usage of ChatGPT or restricting potential use cases (e.g., no code review), put thought into what the potential risks are if your employees are amongst the 100M+ using ChatGPT.
AI exploration can become all-consuming. It is important to establish an internal framework for evaluating opportunities and making sure all substantial investments are tied to an outcome that you’re trying to achieve. Remember that AI doesn’t free you from the demands of ROI.
We’ve highlighted just a few approaches and strategies we’ve heard to make sure your company is best positioned to take advantage of this new wave of AI technology. There is a lot of excitement in the space at the moment, and we believe a lot of the strategy will be about encouraging people to lean in to learn, experiment, and move fast.
If you’re building AI products or have any thoughts on strategies related to incorporating AI into product roadmaps, reach out to us at knowledge@tidemarkcap.com. To learn more about how we think about AI as an opportunity and a threat visit our essay here. If you’d like to get updates as we continue to dive into AI implementation strategies, sign up below.
AI in Practice Series
Part 1: Proprietary Data & Compliance
Part 2: Product Strategy
Part 3: Organizational Change
Stay in the Loop
Enter your details and be notified when we publish new articles on The Highpoint.
